lisa singh 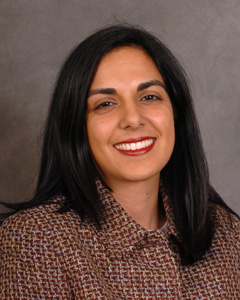
computational & biological inquiry of complex mammalian societies
Overview
Georgetown is currently participating in a unique, interdisciplinary collaborative research project designed to improve the understanding of wild bottlenose dolphin networks. Dr. Mann has been studying wild bottlenose dolphins for over 22 years. Dr. Singh has been working in the areas of data warehousing and data mining for over 10 years. Their goal is to identify new ways to help answer complex biological questions using innovative computer science approaches. Since the inception of this collaboration, others have joined the project team, including Dr. Bienenstock, and the scope of the interdisciplinary collaboration has continued to grow. The research described below is part of the larger Shark Bay Dolphin Research Project, which is becoming one of the world’s most important dolphin research studies world-wide. At Georgetown alone, there are 3 faculty members, 2 full time developers/researchers, 6 graduate students, and 9 undergraduate students working on different aspects of this research.
Motivation
Long-term studies of mammals are precious resources for scientists and the public, but are rarely fully exploited, largely because the data are stored in many formats (e.g. spreadsheets, text, image files), limiting biological inquiry to manual approaches and traditional statistical analyses. At the same time, computer scientists often seek access to large, heterogeneous data sets for developing new analytical approaches for data warehousing queries, data mining algorithms, and knowledge discovery. Mann and
Singh are bridging this gap by developing a data warehouse for the most comprehensive long-term dolphin dataset collected to date. This warehouse, containing 25+ years of observational data, will be used to develop a visual graph inquiry engine that includes a set of dynamic graph query operators, visual analytic tools, and graph mining algorithms to better understand complex, dynamic, multi-relational network data.
Research
We focus on 3 biological questions and 3 computational innovations.
Biological: What are the (1) spatio-temporal and dynamic dimensions of network structure; (2) patterns of socio-cultural transmission of behavior; and (3) social, ecological, and demographic factors influencing female reproduction?
Computational: We are (1) developing a query language and optimized operators for comparing time varying graphs; (2) creating scalable graph mining algorithms for identifying alliances in social communities; and (3) combining a graph database infrastructure and query language with visualization to support visual mining and analytics of large, multi-relational social networks. Our goal is to develop a tool that integrates graph querying, graph mining, and graph visualization to enable both micro and macro analysis and exploration of large animal populations with many measured features. By exploiting both biological and computational approaches, our new tools will help unveil the properties of large, dynamic, heterogeneous datasets and their underlying social complexity.
Broader Impact
This project will serve as a template for large scale animal field studies in the areas of data
collection, data integration, data management, visual data exploration, and data analysis. Few longitudinal datasets on mammals are widely available. Some of the tools we develop will enable scientists to visually explore patterns and data properties directly, which expands beyond the current, and more limited method of downloading variables in table form for traditional data analysis.
Support
In addition to support from Georgetown University, we were awarded 3 collaborative grants to meet these goals. Our NSF-LTREB grant supports the long-term study and database development. The NSF-CDI grant supports innovative computational approaches, specifically visual graph exploration. The ONR grant supports the development of new analytic techniques and algorithms for studying dynamic, multi-modal, multiplexed, multi-attribute social networks with the aim of discovering important features of large unbounded social networks when data are erroneous, ambiguous or incomplete.
highlights
Podcast: Dolphins and Data Unite Unlikely Disciplines (2017)
Nature Article - Social networks reveal cultural behavior in tool-using dolphins (2012)
FinNet - Educational software for children
Shark Bay Dolphin Project Website
332 St. Mary's Building
202-687-9253